Maintenance engineers are constantly aware of obstacles impeding their organization’s maintenance goals. Hence they explore specific actions, activities, and projects that would remove those barriers and support the goals. In the context of their on-going EAM investment, given real day-to-day time and human resource constraints, how can the maintenance department leverage its substantive knowledge and data to achieve physical asset reliability?
They should focus on two general areas or objectives. The first will ensure accurate EAM work order data entry. The technician should reference his observations to a well structured, semantically consistent, universally and frequently accessed, friendly foundation knowledge base that documents failure modes, their effects, consequences, and current consequence mitigating strategies. The second area of interest is the implementation of a continuous systematic decision and knowledge improvement process philosophically integral with daily work order planning, execution, and reporting procedures. These two points of attention will remove the barrier to data driven optimal maintenance decisions.
The well traveled EAM implementation road relies heavily on a hierarchy of failure codes (catalogs) to meet the first of these objectives. But if we were to measure the results to date of the conventional catalog pick list strategy in terms of enabling the typical maintenance organization to achieve reliability from data, they would be dismal.
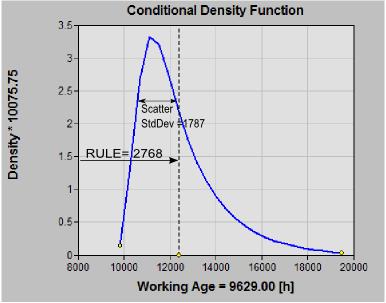
Remaining useful life prediction in EXAKT computed based on age and condition data. Reliability analysis for confident survival prediction is still beyond most maintenance departments due to inaccurate EAM age data.
With regard to the second area of concern, the premise that an EAM catalog process alone as conventionally practiced can assimilate dynamically expanding failure behavior knowledge uncovered and refined almost daily by technicians should be questioned early in the EAM journey. When knowledge embodied in catalog list selections offered to technicians diverges from reality frustration with the system sets in, more so when the technician has no simple mechanism with which to correct the anomaly.
All problems in maintenance decision making as they relate to our desire for continuous, verifiable reliability improvement originate in these two areas. But the complexity of information technology often locks us into procedures that preclude the very activities and goals they are meant to promote.
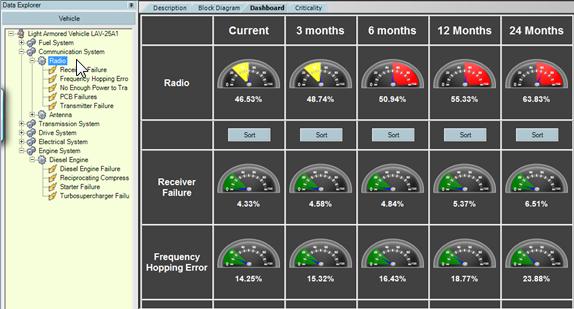
Clockwork Solutions’ SPAR PHM product that unifies Proportional Hazard Models in a Monte Carlo Simulation platform in order to predict reliability knowing current CBM data and alternative maintenance and operational strategies. The dashboard gauges indicate current and projected reliability (survival probability) based on current condition and operational and maintenance plans.
Sometimes we try to exploit existing powerful Reliability Analysis (RA) tools [1] or glimpse ahead at futuristic reliability analytic concepts[2]. In the face of a barrage of technology solutions advanced from a variety of sources the maintenance manager or engineer cultivates a healthy skepticism even regarding those solutions already in the formative stages in the enterprise. That attitude is to be encouraged. It will help you insert timely advice and practical suggestions in the face of overwhelming activities associated with the EAM about to descend, keeping in mind the two major objectives.
© 2014, Murray Wiseman. All rights reserved.
- [1]such as EXAKT, see https://www.livingreliability.com/en/posts/cbm-optimization/↩
- [2]such as SPAR PHM, see exercise 10 at https://www.livingreliability.com/en/posts/cbm-exercises/↩